Artificial intelligence: fully automated processes are the target
By Dr Joachim Hertzberg, Institute for Computer Science, University of Osnabrück
Artificial intelligence (AI) and AI-based robotics have recently received a lot of attention as a future technology - or as a collection of tools currently already in use - in agricultural technology. This applies to statements by machine manufacturers as well as to calls for proposals for applied research projects. To support this claim, one could point to numerous programmatic statements from business and politics. Whether in presentations by international agricultural machinery manufacturers, current programmes of the Ministry of Agriculture or in research funding: artificial intelligence and robotics based on it seem to be "the" technologies of the future in agriculture as well.
Thus, autonomous or fully automated agricultural robots appear almost as a matter of course in discussions about the potential of AI and robotics in a future agriculture. AI software components are of course required to control them. There is no other way to deal with the inevitable effects of uncontrollability and lack of information on arable land in the robot control software.
Artificial intelligence: Where are the practical results?
The practitioners are getting impatient. Because in political strategies for less fertilisation and crop protection, for example, these technologies are already "priced in", so to speak. However, we are still a long way from solutions that can be implemented directly in normal operations. This article therefore does not show any concrete examples of application, but first sorts out the topic: How great is the potential of AI in the first place? What stages of implementation of AI methods in machines and processes in arable farming can be distinguished? And which visions of "agricultural systems of the future" (to quote an earlier BMBF funding programme) with AI contributions are currently "on the way"? To this end, we need to take a quick look at the current state of the scientific field of AI, the relationship between AI and digitalisation, AI-based assistance systems and AI in fully automated ("autonomous") systems.
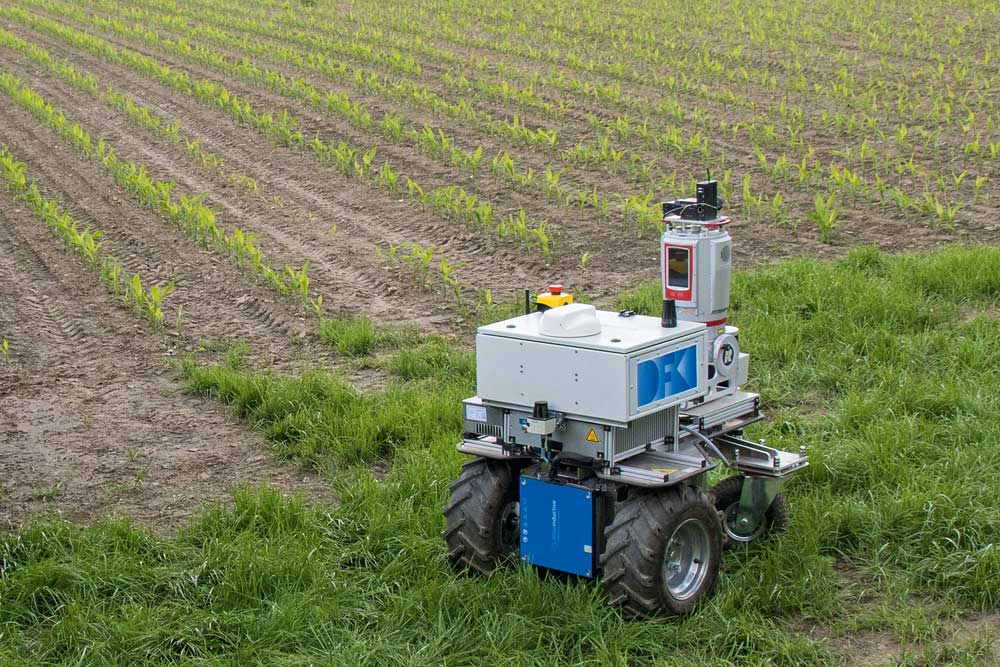
Weak and strong AI: What are we talking about?
The greater the subjective conviction that AI provides powerful tools for building "intelligent" systems, the greater the number of ideas about what exactly AI is. But even Russell and Norvig's AI textbook, probably the most widely used in the world, last reissued in its fourth edition in 2020, does not provide a technical or even formal definition of the field. It does, however, clearly refer to methods for "weak" AI. These are algorithms and formalisms for application-specific goal-oriented control of systems.
The contrast is "strong" AI, which is about simulating human perception and cognition. My own definition goes like this: "AI deals with computer science methods and techniques for building artificial systems that act in a goal-directed manner in environments that are incompletely controllable and/or dynamic and/or known in advance in an imprecise manner". This includes, among other things, methods for problem solving through search, for the logical or also random statistical derivation of knowledge or for the generalisation of general statements from examples.
Machine Learning or Big Data
These two methods have lately gained special attention. This can be explained by recently available very large-volume data sets, very powerful computers and some recent algorithm developments. However, AI is not automatically the same as machine learning! This common equation can be characterised as a fad in view of the history of the field of AI. Such fashions also existed in earlier years, such as the "expert system" wave of the 1970s to 1980s.
Long experience with the development of application systems in environments and problems with dynamics, incomplete control and information shows that only integrated combinations of methods lead to application success. However, a trend from ad hoc methods to those with a deep algorithmic and mathematical foundation is clearly recognisable.
What does AI have to do with digitalisation?
In the Zukunftslabor Agrar of the Centre for Digital Innovations Lower Saxony, we characterise the digitalisation of agriculture as "comprehensive networking and integration of machines, processes and actors within the agricultural value network". This makes it clear that we are talking about something completely different from AI. Nevertheless, AI and digitalisation are often mentioned together and occasionally mixed up.
And this is not entirely out of the world: AI and digitalisation promote each other. The data accumulation that follows from digitisation creates the basis for the application of data-driven methods of AI such as machine learning or general data analysis methods. Conversely, such AI methods allow information and knowledge to be extracted from the data aggregated along the digitalised value chain. These ultimately form the basis for the efficiency and sustainability gains that are promised with digitalisation, especially in agriculture. The distinction between digitalisation and AI is not only of academic interest, but highly relevant in practice. Both realisation must be driven by completely different actors!
A task of product development and practice ...
Digitisation is a challenging topic on several levels: organisational, technical, legal, business management. Large "players" in the agricultural value chain must be persuaded to open up their previously closed systems within clearly defined limits and to let data in, out and through. Small "players" (and here we are also with farmers) must have confidence that their data will be treated with care by all, especially the big ones, and that the potential benefits of data permeability will be distributed fairly across all.
This is a demanding task, but primarily not a research problem for science - apart from detailed aspects of a legal nature, for example. Technically, it is clear how data can flow securely and reliably across different participants: The internet shows us a possible solution every day. Interconnecting through a value chain is not trivial, but there are tried and tested solutions for all information technology issues. In agricultural technology in particular, they are already widely used; think of the Isobus at the bus level and the Agri-Router at the data level. Although the use of these solutions is limited to only a part of the agricultural value chain, the fact that data exchange also works technically between different systems can be observed here in the field every day.
... and a task for research
In AI, the situation is different. Here, in addition to many solutions that are now proven in daily use (speech recognition, navigation systems, recommender systems, gaming software and much more), there are a large number of problems that to date either cannot yet be solved robustly or whose solution with known algorithms and systems still requires expert knowledge about their application. Especially in agriculture, with its sometimes harsh and constantly uncertain application conditions, such problems that cannot yet be solved with certainty are widespread. It still needs some research to realise, for example, robust automatic environment detection of a farm machine on the farm, reliable detection of all weed species on a field or robust optimised automatic control of a harvest campaign.
So what problem does research need to solve? It is about making available AI algorithms and systems for arable farming applications in such a way that they can be used without deep AI knowledge. This is the only way to disseminate knowledge quickly and to validate new AI methods through experience. In order to connect to large volumes of data for AI use, the AI systems should also be operated in the widest possible data "ecosystems". This is one of the goals of the Agri-Gaia project. There we are developing an AI software platform specifically for agricultural applications and also demonstrating it with practical examples. In this way, we want to bring AI developers and agricultural technology manufacturers together in a B2B model. The connection to to a digitalised value chain by connecting to a European data European data ecosystem under development (GAIA-X). We consider and develop AI and digitalisation together, but separate the problems of digitalisation across the value chain from those of developing a domain-specific AI software platform.
On the way to AI-based assistance
Classical processes and/or machines that are typically controlled by humans can be improved through the selective use of AI subsystems. One can imagine a lot under this: Throughput, robustness, standardisability, user-friendliness or combinations of these. In other words, it is about the further development of machines and processes, not future systems. This has obvious advantages over revolutionary changes: Clear boundary conditions for the innovations, quick implementability, better acceptance and calculable market conditions. In agriculture, too, such AI-based assistance components are currently being developed with great vigour. Agriculture with its many uncertainties (weather, soil, animals, market prices and more) fits AI. But "how much percent AI" will be in an agricultural machine with AI-based assistance functions, and how do machines and processes change?
How much computer science is in an agricultural machine?
How much materials engineering? How much physics? These are obviously nonsensical questions. It is clear that today's machines are inconceivable without computer science, and neither are they conceivable without materials technology and physics. Combine harvesters existed before computer science, and today's are better than those without. Incidentally, the process of mowing and threshing also existed before combine harvesters. This line of technological development continues when AI modules become available, for example for machine learning or automated process planning: The machines and the processes will become better - if they don't, these modules will not be saleable. It is not about AI as such, but about machines and processes with higher benefits. In fact, we are already seeing this in some products, such as the evaluation of camera images in real time.
Conclusion
AI in the sense of computer science methods for dealing with uncertainty in a targeted way fits well with digitalisation. And AI fits well with agriculture - it offers perfect opportunities for agriculture, which is already highly digitised. This realisation has arrived in agricultural technology as well as in research. Current research and development activity is correspondingly lively. In the short and medium term, AI will contribute to the construction of assistance systems for agricultural machinery and agricultural processes. It can play an important role in the development of new agricultural systems - but only if it is considered from the outset in the overall picture of these new systems.
(Revised version of a contribution to the KTBL Days 2021)